Artificial intelligence is helping improve climate models
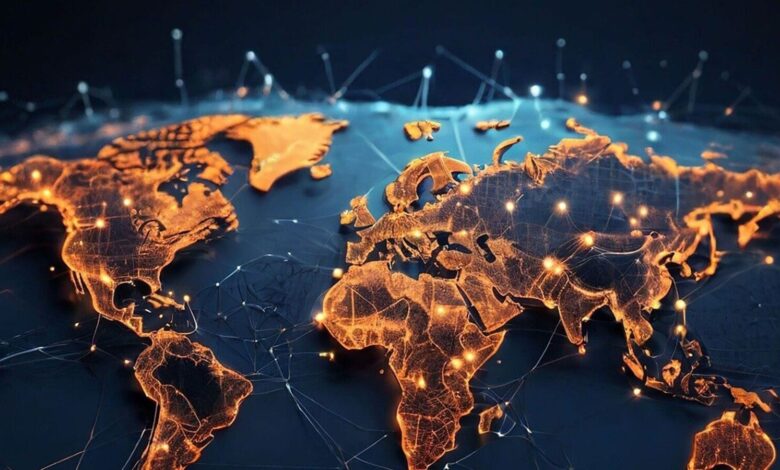
No model is perfect. Those modeling climate trends and impacts are forced to exclude many things, either because the underlying scientific processes are not yet understood or because representing them is too computationally expensive. This results in significant uncertainty in the results of the simulation, which falls in line with real-world results. For example, the main battle among delegates in Baku will be over how much money should be given to poor countries to help them decarbonise, adapt or recover. The amount needed for adaptation and recovery depends on factors such as sea-level rise and seasonal changes, which climate modelers are still struggling to predict with much certainty. As interactions become more specific, more accurate estimates will become more important.
The models that matter most in such discussions are run as part of the Coupled Model Intercomparison Project (CMIP), an initiative that coordinates more than 100 models produced by about 50 teams of climate scientists around the world . They all try to approach the problem in the same way: first using equations representing physical processes to predict what the conditions might be in each cell and how they might change over time. Dividing the world and its environment into a grid of cells.
When CMIP began in 1995, most models used cells hundreds of kilometers wide – meaning they could make useful predictions about what might happen to a continent, but not necessarily differently. For different countries. Halving the size of cells requires approximately ten times more computing power; Today’s models, thousands of times more powerful, can simulate cells of about 50 km per side.
Clever computational tricks can make them even more detailed. They have also become better at representing the detailed interactions that occur between the atmosphere, oceans, and land – such as how heat flows through the oceans or how soil moisture changes with temperature. But many of the most complex systems remain elusive. For example, clouds pose a serious problem, because they are too small to be captured in 50 km cells and because even small changes in their behavior can lead to large differences in projected levels of warming.
Better data will help. But a more immediate way to improve climate models is to use artificial intelligence (AI). Model-makers in this area have begun to boldly claim that they will soon be able to overcome some of the resolution and data problems faced by traditional climate models and also get results more quickly.
Google engineers have been the most optimistic. NeuralGCM, the company’s leading AI weather and climate model, has been trained on 40 years of weather data and has already proven itself to be as good at predicting weather as the models with which these data were originally compiled. Were done. In a paper published in Nature in July, Google claimed that its model would soon be able to make predictions over longer time scales faster and using less power than existing climate models. With additional training, the researchers also believe that NeuralGCM will be able to provide greater certainty in important areas such as changes in monsoons and tropical cyclones.
The researchers say this optimism comes from the unique capabilities of the machine-learning tool. Where existing models approach difficult physics problems using approximations, NeuralGCM’s creators claim it can be guided by detecting patterns in historical data and observations. These claims seem impressive, but have not yet been evaluated. In a preprint posted online in October, a team of modelers at Lawrence Livermore National Laboratory in California noted that NeuralGCM will be limited until it incorporates more physics to play on the ground.
Others are more skeptical that AI methods used in short-term weather forecasting can be successfully applied to climate. “Weather and climate are both based on physics, but present different modeling challenges,” says Gavin Schmidt, a climate scientist who runs NASA’s Goddard Institute for Space Studies. For one thing, the available data are rarely of the same quality. For weather prediction, large amounts of excellent data are generated every day and, therefore, able to continuously validate the previous day’s predictions, climate models do not enjoy the same luxury, moreover, they have to be saw Faces the challenge of simulating more extreme conditions than any that has occurred over a period of days rather than centuries.
Yet AI can help improve climate models by addressing another major source of uncertainty: human behavior. So far, this has been overcome by codifying different social and political options into sets of fixed scenarios that can then be modeled. This method makes evaluation possible, but is inflexible and often vague. With the help of AI, existing tools known as emulators can adapt traditional models to suit the needs of their end users. Such emulators are now used by cities to plan infrastructure projects, by insurers to assess risk, and by agricultural companies to predict changes in crop yields.
Unlike models like Google’s NeuralGCM, which are trained on the same weather data as today’s top climate models, emulators are typically trained on the output of full-scale climate models. This allows them to improve the models themselves – both the new physics they are able to model and the way they extrapolate beyond historical data. For example, one such emulator, developed by the Commonwealth Scientific Industrial Research Organization in Australia in 2023, was able to adjust predictions about future emissions levels one million times faster than the model on which it was trained.
Reducing uncertainties in climate models and, perhaps more importantly, making them more widely available, will increase their usefulness to those facing the complex challenge of tackling climate change. And hopefully that will mean better feedback.
© 2025, The Economist Newspaper Limited. All rights reserved. From The Economist, published under license. Original content can be found at www.economist.com
(TagstoTranslate)artificial intelligence(T)COP29(T)climate models(T)climate change(T)AI(T)sea level rise(T)seasonal change(T)coupled model intercomparison project(T)climate scientists(T) NeuralGCM(T) climate models using artificial intelligence
#Artificial #intelligence #helping #improve #climate #models